Atoms and Molecules on Surfaces Explored by Machine Learning
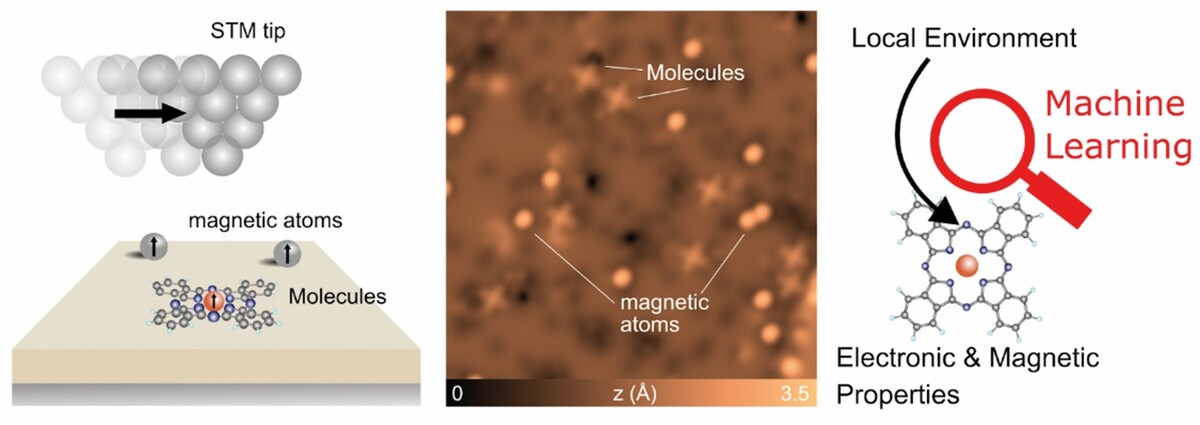
High-resolution scanning tunneling microscopy (STM) constitutes one of the best ways to access the nanoworld. In simple terms, it is an atomic sized finger that can scan a
sample surface with atomic precision. Thus, it can identify individual atoms and molecules on top of a surface and determine their electronic and magnetic properties. However, finding connections between property variations and the individual local environment is cumbersome. Moreover, STM operation is still a time-consuming effort and requires skilled students and experienced researchers. With this challenge in mind, Philip reached out to Pascal. The idea for the YIN grant was born: a machine learning assisted, systematic analysis of data generated by STM techniques would offer the opportunity for automation and speed-up. In addition, it has the potential to unravel new physics by utilizing the additional leverage of machine learning (ML). The project combines the expertise of Philip Willke and Pascal Friederich that is of high-resolution low-temperature STM on the one side and ML methods on the other. Philip’s team took datasets from a system, studied in detail with a great variety of STM techniques (see picture; comp. X. Zhang et al., Nat. Chem. 14, 2022), which constitutes a well established model system for the application of ML techniques.
While on the atomic scale each molecule or atom is technically identical to others of the same kind, the local environment – geometric and electronic is never the same. As shown in the picture, local defects or strains in the substrate might change the physical properties of the individual molecules or atoms in the vicinity. Finding connections
and identifying the hidden physical laws in the data is difficult for human operators: Here lies the potential for MLbased data processing and data acquisition.
Since the start of the project, Philip and his team generated a large amount of high-quality STM data of molecules and atoms on surfaces. The data was transferred to the team of Pascal, where machine learning models were trained to identify and extract single objects on surfaces. Object detection models, widely used in computer vision, were used for this task. Two bachelor theses have already been finished on this project. The students developed protocols of image recognition and task automation using ML and deep learning on neural networks.
"In the next step, we plan to use unsupervised learning methods to identify groups of similar objects that are detected in the images. We expect the majority groups to be in different oxidation states of iron atoms as well as the FePc molecules. Though, there might be additional objects that occur frequently, which remain to be identified. Once the objects can reliably be detected, we plan to correlate their local environment to magnetic properties measured in Philip’s group," says Pascal Friederich.